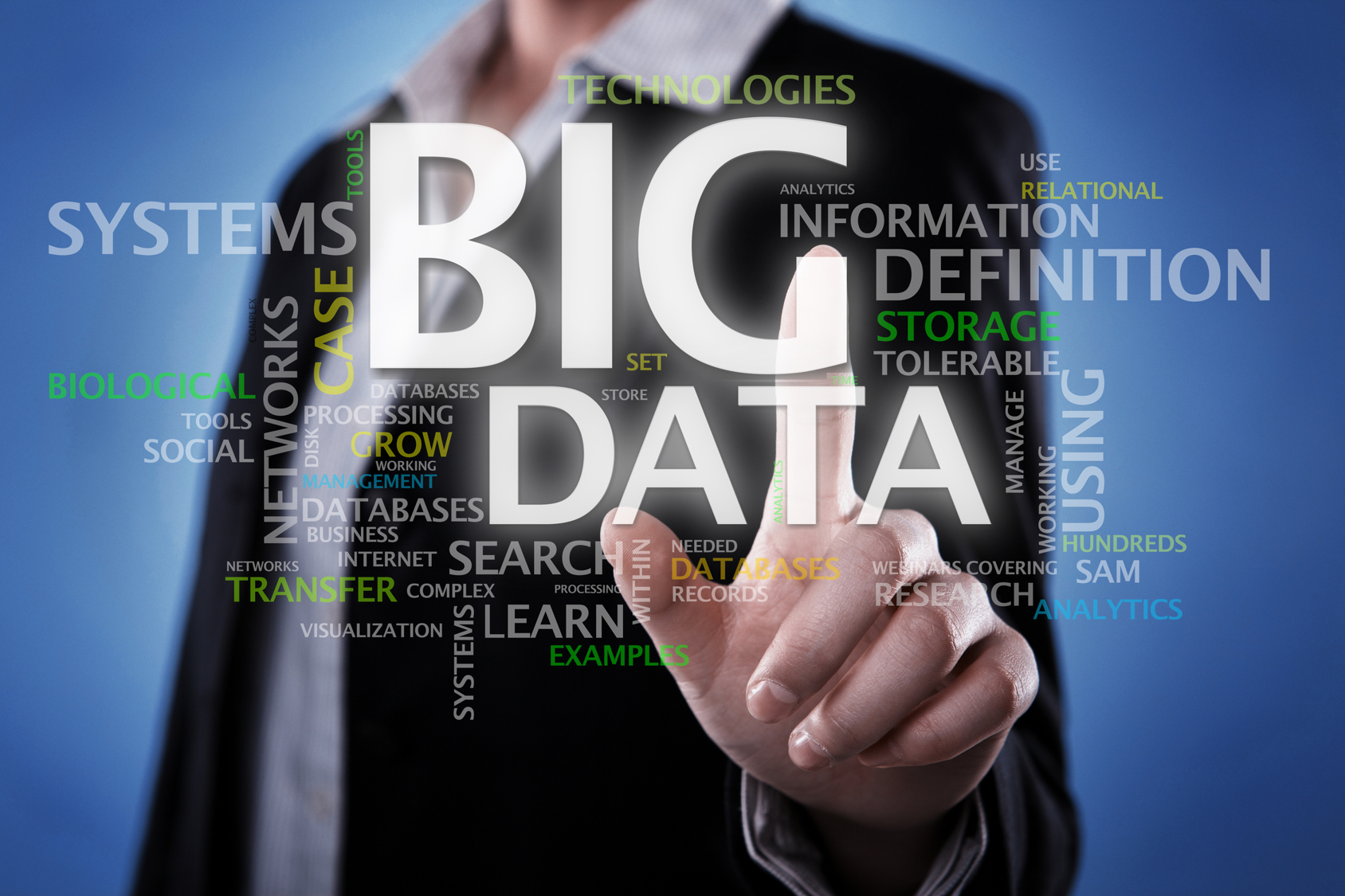
BIG DATA
Introduction:
Big data refers to extremely large and complex datasets which traditional data processing software cannot adequately handle. The act of analyzing these massive volumes of data is referred to as big data analytics. The core challenge with big data is turning vast volumes of messy, unstructured data into meaningful insights and predictions.
Characteristics of Big Data:
There are 5 main characteristics used to define big data known as the 5Vs:
Volume: This refers to the vast amounts of data generated in every second. Social media, smartphones, IoT devices and more are generating exabytes and zettabytes of data.
Velocity: Data is often generated extremely fast, that it needs to be analyzed and processed in real-time. RFID tags, sensors and smart meters are always generating new data.
Variety: Big data comes in many formats including structured numeric data as well as unstructured text, video, audio and financial transactions. All forms of data have potential value.
Veracity: Big data can often incomplete, inaccurate and inconsistent requiring analytics techniques to clean and transform datasets.
Value: The most important characteristic – the ability of big data analytics to unlock powerful insights that can transform products, services, and operations.
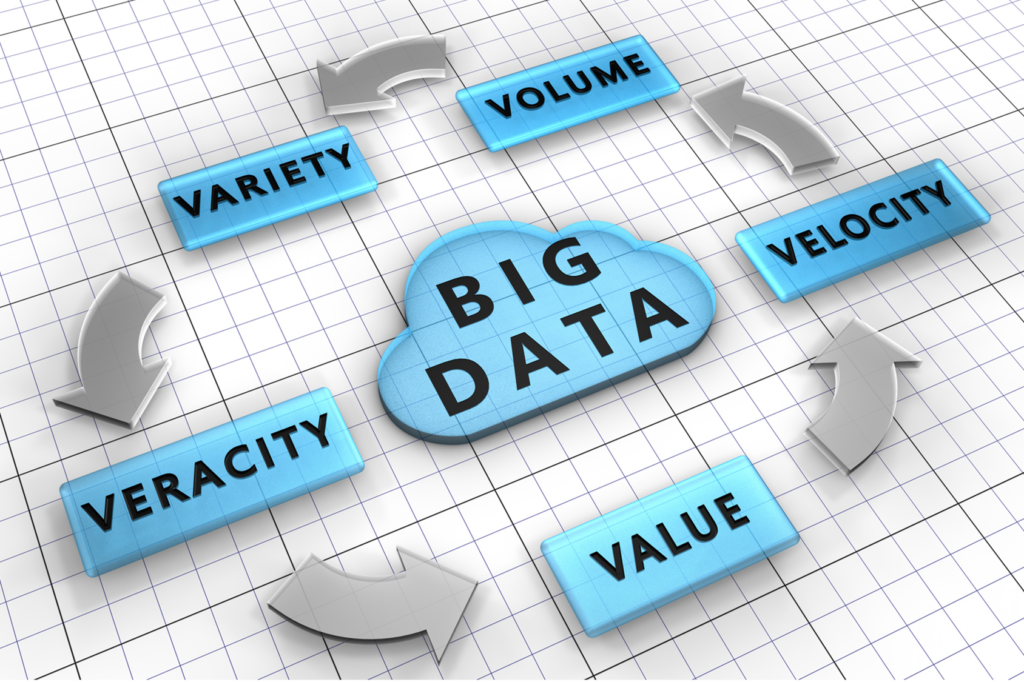
Sources of Big Data:
Big data comes from an incredible variety of sources:
Social Media: Facebook posts, Tweets, Instagram photos, YouTube videos and more.
Commercial Transactions: Online purchases, credit card payments, product reviews, loyalty card records.
Sensors: IoT devices, equipment sensors, cameras, microphones, RFID readers and more.
Science & Research: Genomics, biometric research, scientific studies & experiments.
Mobile & GPS: Cell tower signals, phone call logs, text messages, mobile apps.
Server Logs & Networks: Server activity logs, network traffic data, Wikipedia edits
Big Data Analytics Process:
The process for obtaining value from big data involves:
Data Acquisition
Information Extraction and Cleaning
Data Integration
Data Modeling
Exploratory Data Analysis
Predictive Analysis and Machine Learning
Once these steps have been completed additional steps can be taken to visualize key findings to help guide business strategy and decisions.
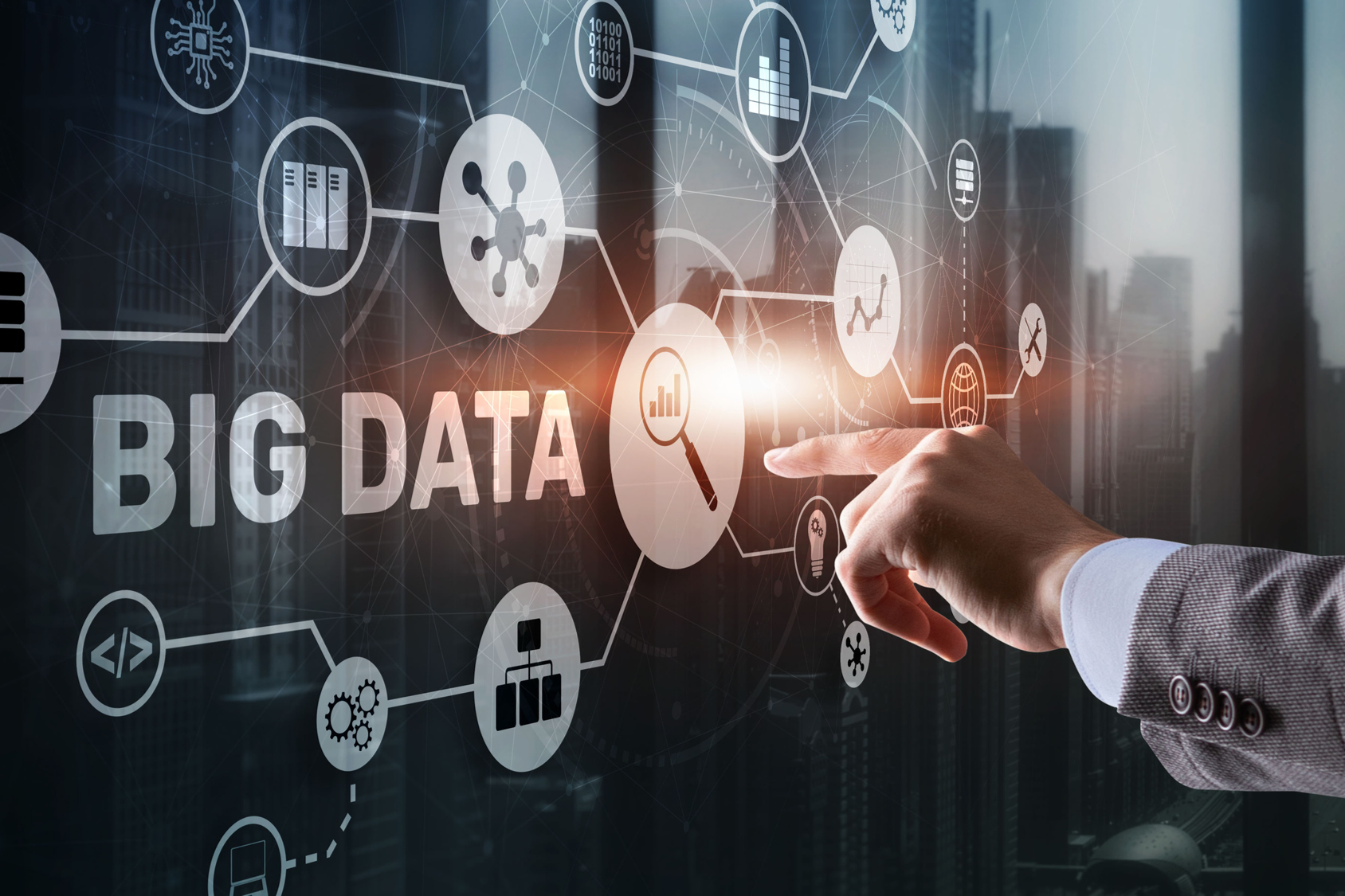
Big Data Technology Landscape:
Critical technologies used for big data analytics include:
Hadoop
Spark
Kafka
NoSQL Databases
Data Lakes
Machine Learning
Cloud Computing Environments
The Importance of Big Data:
Big data analytics helps organizations in industries ranging from healthcare to manufacturing optimize operations, enhance offerings, reduce costs and risks. It is becoming an indispensable asset in the modern data-driven global economy. The future is likely to see big data grow, even bigger in importance.
Characteristics
of Big Data:
There
are 5 main characteristics used to define big data known as the 5Vs:
Volume: This
refers to the vast amounts of data generated in every
second. Social media, smartphones, IoT devices and more are generating exabytes
and zettabytes of data.
Velocity: Data
is often generated extremely fast, that it needs to be analyzed and
processed in real-time. RFID tags, sensors and smart meters are always
generating new data.
Variety: Big
data comes in many formats including structured numeric data as well as
unstructured text, video,
audio
and financial transactions. All forms of data have potential value.
Veracity: Big
data can often incomplete, inaccurate and inconsistent requiring analytics
techniques to clean and transform datasets.
Value:
The
most important characteristic – the ability of big data analytics to unlock
powerful insights that can transform products, services, and operations.